Delving Deep into Hybrid Annotations for 3D Human Recovery in the Wild
Abstract
Though much progress has been achieved in single-image 3D human recovery, estimating 3D model for in-the-wild images remains a formidable challenge. The reason lies in the fact that obtaining high-quality 3D annotations for in-the-wild images is an extremely hard task that consumes enormous amount of resources and manpower.
To tackle this problem, previous methods adopt a hybrid training strategy that exploits multiple heterogeneous types of annotations including 3D and 2D while leaving the efficacy of each annotation not thoroughly investigated.
In this work, we aim to perform a comprehensive study on cost and effectiveness trade-off between different annotations.
Specifically, we focus on the challenging task of in-the-wild 3D human recovery from single images when paired 3D annotations are not fully available.
Through extensive experiments, we obtain several observations: 1) 3D annotations are efficient, whereas traditional 2D annotations such as 2D keypoints and body part segmentation are less competent in guiding 3D human recovery. 2) Dense Correspondence such as DensePose is effective. When there are no paired in-the-wild 3D annotations available, models exploiting dense correspondence can achieve 92% of the performance compared to a model trained with paired 3D data.
We show that incorporating dense correspondence into in-the-wild 3D human recovery is promising and competitive due to its high efficiency and relatively low annotating cost. Our model trained with dense correspondence can serve as a strong reference for future research.
Framework
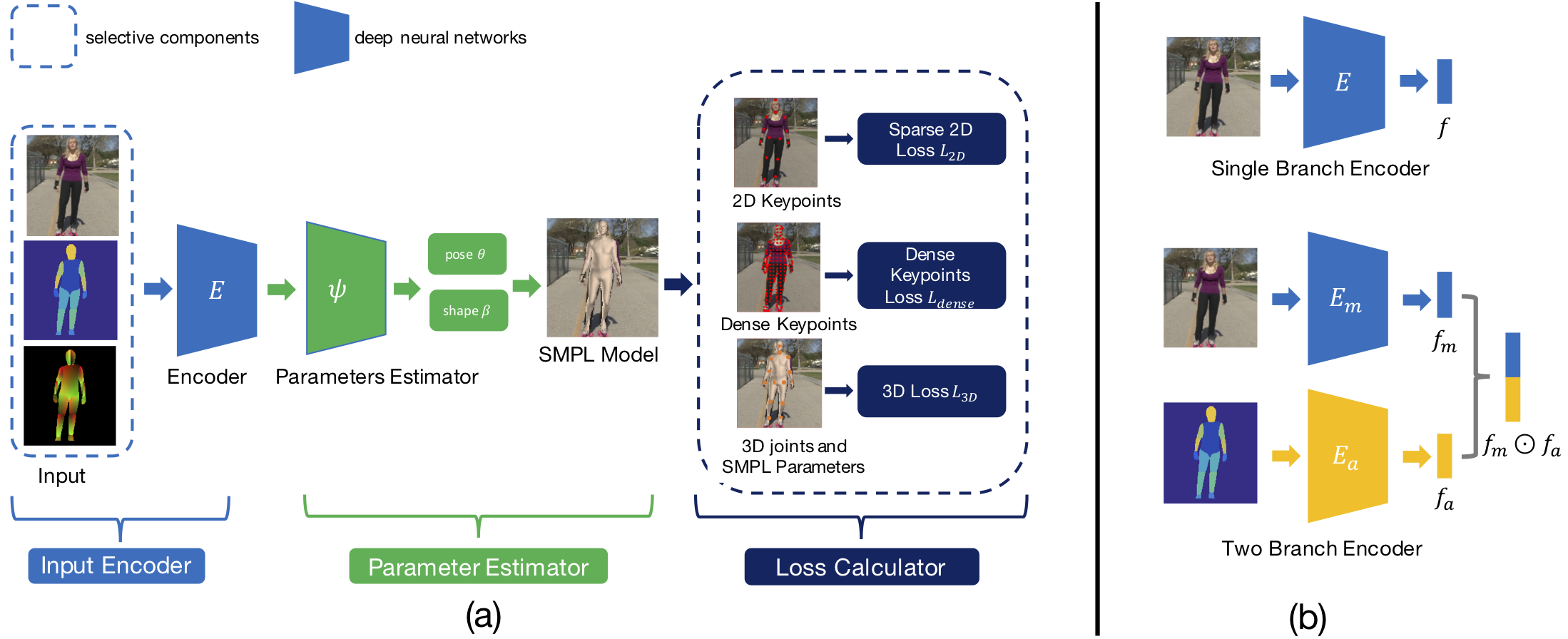
Our framework is composed of three components. 1) Input encoding part takes inputs and outputs encoded features. 2) Parameter estimator estimates the pose and shape parameters of the SMPL model given the outputs of the encoder. 3) Given estimated parameters, SMPL model generates predicted 3D joints, 2D keypoints and dense keypoints to calculate loss. Figure (b) shows two possible architectures of the input encoder. Input encoder could either be composed of a single branch that only takes one kind of inputs or two branches that takes original images and the other auxiliary inputs.
Experimental Results

The evaluation metrics are PVE, MPJPE and PVE-T, separately. For all metrics, lower is better. "3D" refers to paired in-the-wild 3D annotations. "20% 3D" refers to 20% randomly selected 3D annotations. "Sparse 2D" refers to sparse 2D keypoints. "Dense" refers to dense correspondence, namely, IUV maps generated by DensePose.
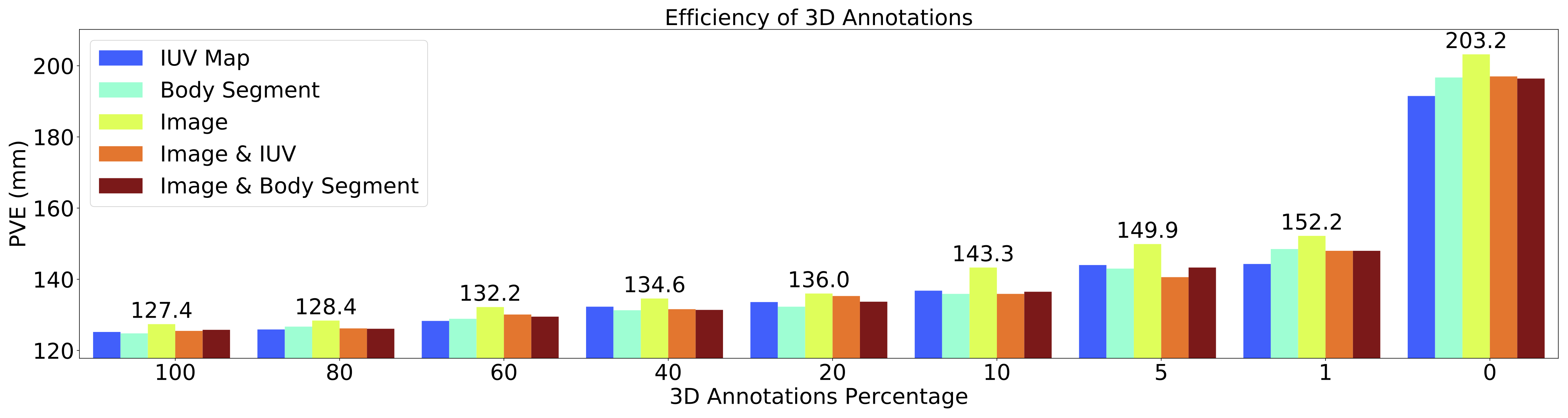
Visualization
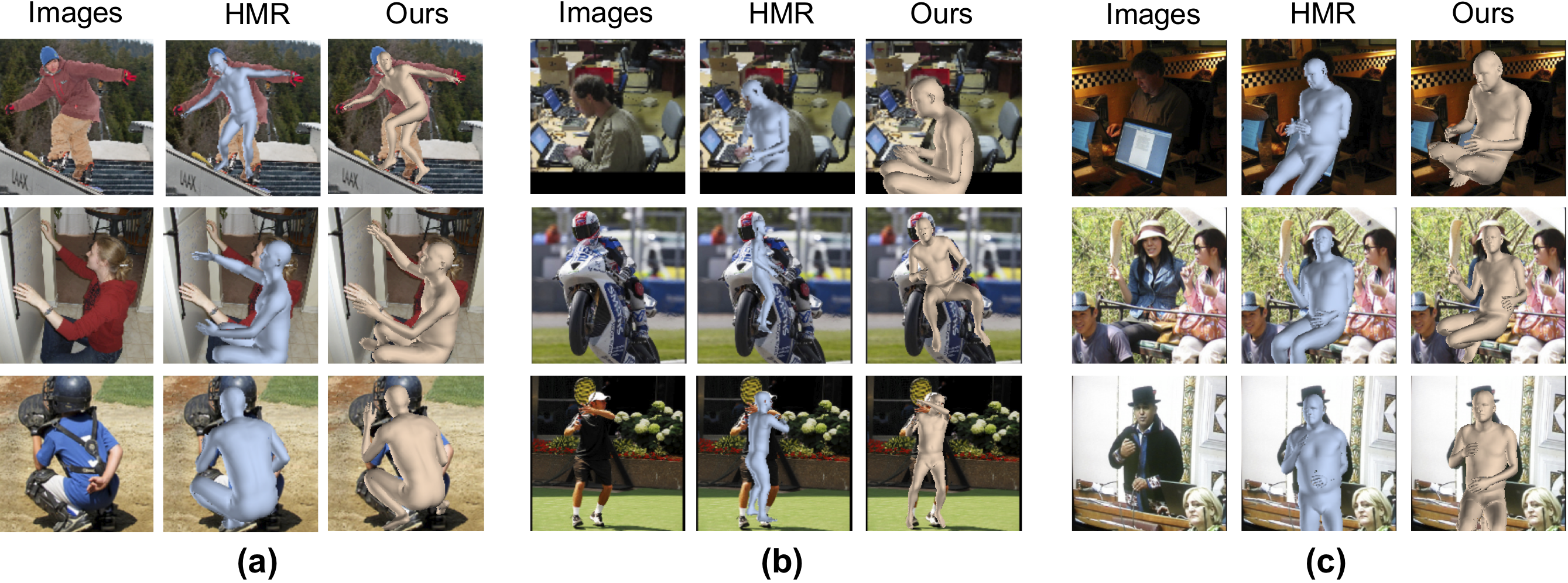
Results of our flow-guided video inpainting approach. For each input sequence (odd row), we show representative frames with mask of missing region overlay. We show the inpainting results in even rows.
Materials
Citation
@InProceedings{Rong_2019_ICCV, author = {Rong, Yu and Liu, Ziwei and Li, Cheng and Cao, Kaidi and Loy, Chen Change}, title = {Delving Deep into Hybrid Annotations for 3D Human Recovery in the Wild}, booktitle = {Proceedings of the IEEE international conference on computer vision}, year = {2019} }